German AI case-law: Disclosure of the structure of AI networks
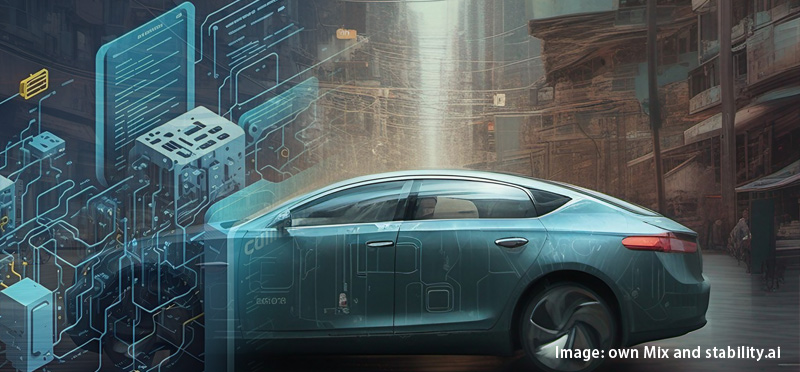
Even if the fictitious skilled person would use methods from the field of machine learning as well as AI networks and time series analysis, it is obvious that the indications "training data" and "engine control unit" are not process steps.
This is a finding from the decision 19 W (pat) 32/20 on the disclosure of the structure of AI networks of the German Federal Patent Court (BPatG), and it is an important aspect for patent applications with AI applications: AI inventions must be sufficiently disclosed, too, and that is not easy.
What this would look like in practice is shown by the decision of the Federal Patent Court discussed here on the patent application entitled "Method for determining and predicting the individual oil change interval of an internal combustion engine".
The facts
According to the patent application, the invention is based on the task of creating a method that is intended to determine the oil life for the respective internal combustion engine with any individual load or use, while avoiding rigid worst-case intervals.
Basically, known techniques and test systems are used, the patent application mentions among others
- the NRTC test (a standardised, dynamic transient test cycle for mobile machines and devices on the test bench (Nonroad Transient Cycle (NRTC))
and
- the time series analysis in combination with exponential smoothing (a common standard method of analysis for trend extrapolation).
Patent claim 1 according to the main request is intended to protect a method for predicting and indicating the individual oil change interval of an internal combustion engine. However, in the opinion of the Federal Patent Court as well as the examining division of the German DPMA, this was not sufficiently disclosed.
However, according to the BPatG, the following questions in particular remained open and were not sufficiently disclosed:
How is the prediction of the individual oil change interval made possible?
And what is the structure of the AI networks for this purpose?
The calculation of the prognosis
Even if the person skilled in the art understands the chronological sequence of oil sampling and analysis for determining the wear indicators in accordance with the features of the patent application in certain scaling, which is ultimately used to calculate the oil change interval, the BPatG considers that the question remains open as to what it means that the cycles are scaled in time.
The calculation for the prediction could also not help in the realisation of the claimed method.
Equation 6, for example, a slope equation for the forecast after how many hours the upper threshold of oil wear is reached, contains the factor length(Forecast vec) in the denominator.
However, according to the BPatG, it was obvious to the skilled person that this vector could not mean an element of a vector space of linear algebra or analytical geometry, but at most the vector concept of a so-called n-tuple of real numbers motivated by the coordinate representation of geometric vectors.
The expert could understand length as the number n of elements contained in the tuple - but that is excluded here, since the vector "Forecast vec [%]" was characterised as dimensionless and assigned a length that explicitly has the dimension of a time ("FCH [h] ... = 48 h...").
Overall, the calculation for the prediction was only a supposedly detailed instruction in the patent application, the BPatG found. Feature M1.4, according to which "modelling and implementation in the engine control unit" is to take place, was incomprehensible in two respects and thus not comprehensible.
Structure of the AI networks - insufficiently described
It is explained in the patent application that the respective "measured" damage indicator is the "target variable" of an artificial neural network (artificial intelligence (AI)) into which the influencing variables are integrated or averaged and input in summary form.
With regard to the artificial neural networks on which the degradation model is based, the expert learns that these are optimised by heuristic algorithms, adapted to the wear behaviour of the damage indicators and the load cycles are modelled by training the algorithms in the engine control unit.
However, the structure of the AI networks is not described in the application. The BPatG found fault with the fact that it was neither stated how many artificial neurons were located on how many layers (single-layer or multi-layer, hidden layers), nor how they were connected with each other (e.g. feedforward, convolutional or recurrent networks).
What is meant by training the AI?
The training data for the topology and structure of the AI also raises questions rather than providing comprehensible instructions for a specialist.
According to the patent application, the training data is composed of the measured integral influence variables over the respective cycle period and the course of the wear indicators of the oil. All influence quantities would be integrated or averaged and combined into the input vector of the artificial neural network training data required for supervised learning, with the target quantity being the respective measured (oil analysis) -damage indicator.
But, it remains open whether the "measured" damage indicators given as target values are the output values in the training data and whether and, if so, how the wear indicators are used in the process. And what is meant by a damage indicator at all? The expert could only guess.
The BPatG said that the description in particular contained a large number of erroneous, unclear or contradictory statements and, moreover, contained neither an example of an embodiment nor a technical teaching that would make at least one variant of the method accessible to the skilled person. The invention in the application is not disclosed so clearly and completely that a person skilled in the art can carry it out (§ 34 (4) German Patent Act).
Indeed, it is not necessary for a patent claim to contain all the information necessary for carrying out the invention. However, a general solution scheme must be provided, and this must then be elaborated in the description or in embodiments with the necessary details.
Our patent law firm has deep experience in this field, especially in patent protection for AI-involved inventions. Please contact our law firm Köllner & Partner, an enquiry is free of charge and without obligation. You can reach us by phone at +49 69 69 59 60-0 or please send us an email info@kollner.eu.